www.magazine-industry-usa.com
30
'19
Written on Modified on
The role of machine vision in manufacturing
Machine vision already makes an important contribution to the manufacturing sector, primarily by providing automated inspection capabilities as part of QC procedures.
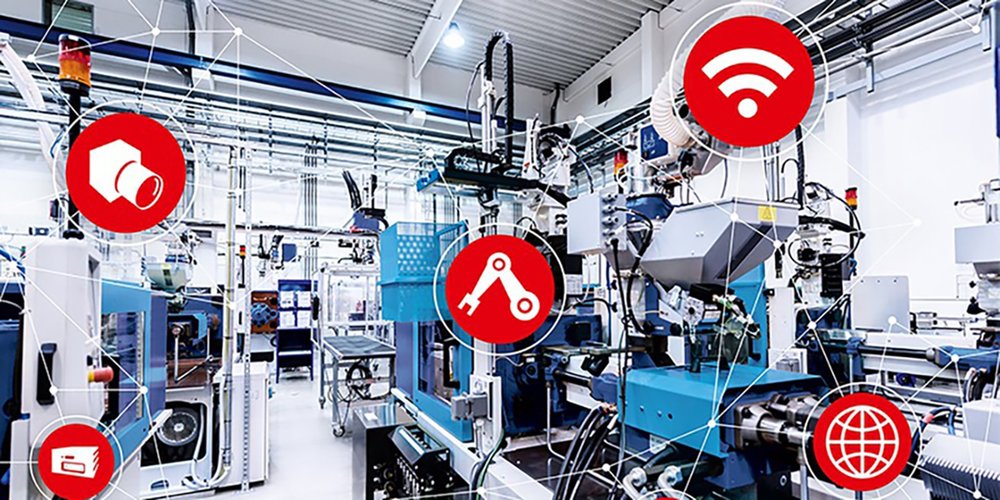
An enabling technology
With rapid developments in many different areas including imaging techniques; CMOS sensors; embedded vision; machine and deep learning; robot interfaces; data transmission standards and image processing capabilities, vision technology can benefit manufacturing industry at many different levels. New imaging techniques have provided new application opportunities.
For example hyperspectral imaging can provide information about the chemical composition of the materials being imaged. Computational imaging allows a series of images to be combined in different ways to reveal details that can’t be seen using conventional imaging techniques. Polarisation imaging can display stress patterns in materials.
Other developments in machine vision technology lead to enhanced performance, integration and automation in manufacturing industry. The degree of integration can range from manual assembly assistance through to complete integration into OEM equipment and on to the demanding requirements of Industry 4.0.
Aiding manual assembly
There are still huge numbers of products that are assembled manually and a ‘human assist’ camera can be used to help to prevent errors in such operations. The operator follows a set of assembly instructions loaded into the camera and displayed on a monitor.
After every action the system compares the result to the correct stored image to ensure that it has been carried out correctly and completely before the operator can move on to the next step. If an action is incomplete or if a mistake is made, it is displayed to the operator so that it can be corrected. Each step completed can be verified and recorded to provide data that can be used for assembly work analysis and traceability.
Adding vision to the production line
Using vision inspection on a manufacturing or packaging line is a well-established practice. Systems range from single-point self-contained smart cameras that carry out an inspection task and deliver a pass/fail result to the control system, to PC-based systems that may feature multiple cameras and/or multiple inspection stations. Vision systems can be retrofitted to existing lines or designed into new ones.
Vision inspection can also be used in conjunction with statistical process control methods to not only check critical measurements but to analyse trends in these measurements. In this way, interventions can be made to adjust the process before any out-of-tolerance product is produced. This is probably the closest forerunner to the requirements of Industry 4.0.
Vision-guided robots
Industrial robots are already used extensively and with the emergence of collaborative robots and rapid developments in 3D image processing, they are being used much more in combination, particularly for vision-guided robotics.
The vision system identifies the precise location of the object and these coordinates are transferred to the robot. Massive strides in vision-robot interfaces make this process much easier. One of the most popular uses for 3D robotic vision is in pick and place applications.
Embedded Vision
The availability of small, embedded processing boards, usually based on ARM architecture, offers great potential for the development of vision systems embedded into other equipment and manufacturing processes. Many of the leading image processing libraries and toolkits can now be ported to these platforms, offering a wider range of vision solutions in this format.
Combining these processing capabilities with low cost cameras, including board level cameras, means that vision systems could be incorporated into a wide variety of products and processes with comparatively small cost overheads.
Machine and deep learning
There has been a lot of hype about deep learning in machine vision, which uses convolutional neural networks (CNNs) to carry out classification tasks by identifying characteristics learnt from a set of training images. However, the challenge remains that in industrial applications the number of available training images is limited while the tools, training time and processor resources remain high.
Other machine learning approaches are rapidly becoming recognised as a cheaper and simpler to implement alternative to deep learning for industrial applications. This is likely to find traction for high-performance, flexible vertical solutions that will even run on inexpensive embedded systems, making extremely cost-effective systems possible.
Onwards to Industry 4.0
The essence of the smart factory of the future is to optimise the process using big data analytics based on the feedback from many different types of sensors that are monitoring the process. These, of course, will include simple and smart vision sensors as well as more sophisticated vision subsystems or systems. Critically, Industry 4.0 requires a common communication protocol for all sensor types in order to allow data transfer and sharing.
One standard which is proving popular in this area is the OPC UA platform-independent, open standard for machine-to-machine communications. Recently the VDMA (the Mechanical Engineering Industry Association in Germany) has announced OPC UA Companion Specifications for Robotics and Machine Vision which will provide compatibility with this standard for robots and vision systems respectively. The building blocks are beginning to come together.
www.stemmer-imaging.com