www.magazine-industry-usa.com
04
'20
Written on Modified on
DataProphet
Consistent data is key to AI process optimization
The consistency of a factory’s data is crucial to unlocking the long-term productivity benefits of Artificial Intelligence (AI), according to DataProphet, a global leader in AI for manufacturing.
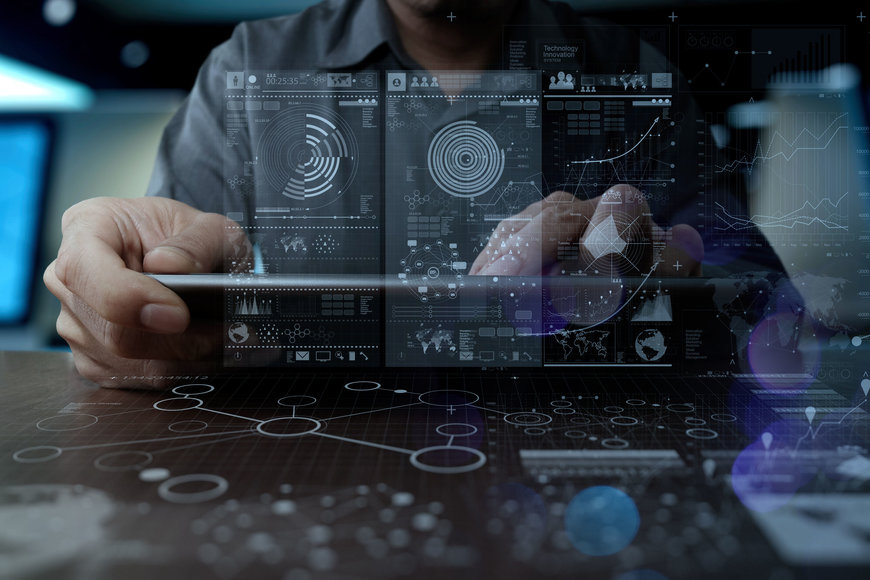
Today's state-of-the-art AI algorithms learn complex patterns from historical process data. This task typically requires that thousands of historical production cycles be available in the data. More data tends to result in further improvements.
In addition, fresh process data needs to be regularly available to the AI pipeline in order to keep the AI model current with factory operating conditions.
These requirements for sufficient, recent, and regular data, however, are incomplete if an industrial AI deployment does not guarantee the consistency of the digital representation. This consistency, in turn, depends on appropriate methods for recording and collecting process data.
Joris Stork, a Senior Data Scientist at DataProphet, has commented on this point: “Continued data availability goes hand in hand with the requirement for data consistency. However, errors can occur if a factory intermittently changes the representation of variables in key data exports, such as whether a three-state indicator is represented as a number from the set 1, 2, 3 or as a string of text from the set 'red', 'orange', 'green'. If uncaught, these types of changes could quietly corrupt the optimisation model and potentially result in a negative impact on process quality. We’ve got a few good ways to keep this in check.”
“The digitisation and automation of process data infrastructure and data exports goes a long way towards addressing these issues. Whatever the factory's data infrastructure, a good AI ingest pipeline should feature a robust data validation layer, to ensure inconsistencies are flagged and fixed.”
Manufacturers sometimes overlook the importance of consistent data representations, as they seek to maximize data volume and data coverage. In fact, these data requirements must be addressed together as a package in order to open the door to AI optimization.
Mr Stork adds: “One of the most common questions we are presented with is, how many rows, i.e. production examples, make a sufficient training set? The answer depends on the complexity of the process. The sample needs to be a sufficient representation of this complexity. In the manufacturing context, the lower bound typically ranges from a few hundred to several thousand historical examples. Training a model on more data than is strictly sufficient, however, tends to increase the model's confidence and level of detail, which in turn is likely to further improve the optimisation outcome.”
DataProphet is one of the global leaders in AI for manufacturing. DataProphet PRESCRIBE is a unique deep learning solution that prescribes optimum plant control parameters, frequently reducing the cost of non-quality by more than 50 percent, through a customized single model approach, while taking into account higher-order effects.
For more information on DataProphet and its Artificial Intelligence Suite, please visit: www.dataprophet.com.
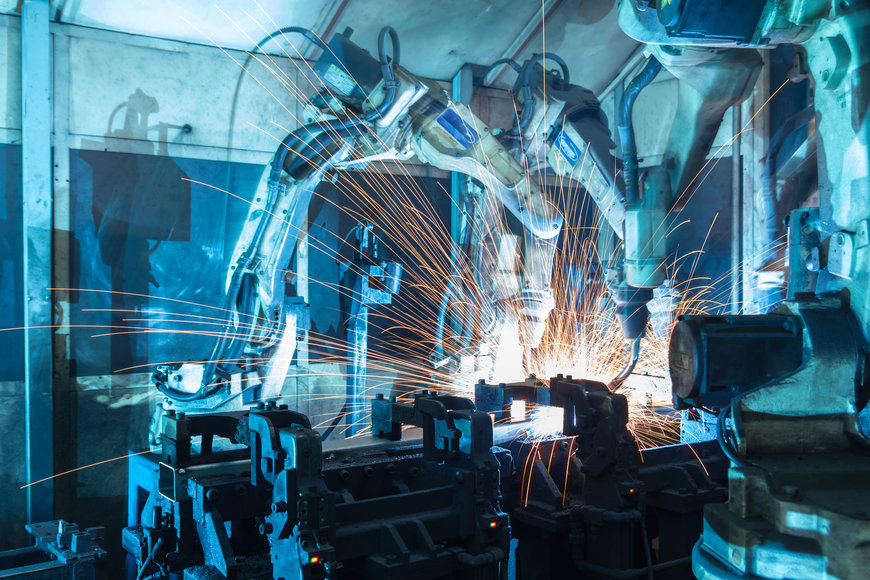
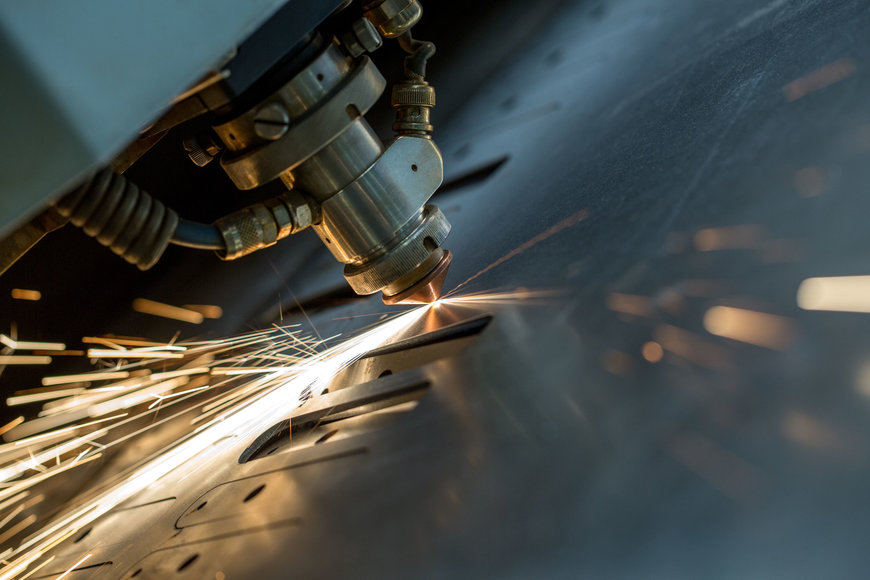